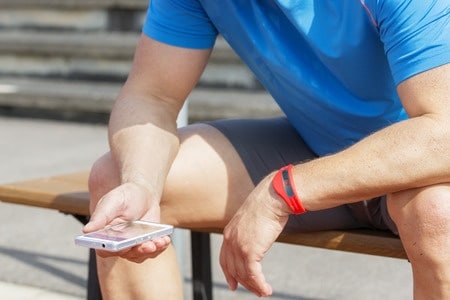
Heart rate variability (HRV) is an important marker of an individual’s physiological stress level. When properly analyzed in the context of training, it can be key in helping athletes and sport enthusiasts monitoring training load and further optimizing performance.
Given the technology available today, HRV can be computed accurately without the need for any external sensor or device.
How Does HRV Work?
Our heart rate is governed by many variables.. including our nervous system. Our nervous system has many working parts. The autonomic nervous system is but one of them. Our cardiovascular system is mostly controlled by autonomic regulation through the activity of sympathetic and parasympathetic pathways of the autonomic nervous system. Analysis of HRV enables us to understand this control mechanism. Several studies on HRV highlighted how different features can provide insights on autonomic regulation and especially on parasympathetic activity [1, 2], the branch of the nervous system mainly responsible for the body’s resting functions.
Simply put, monitoring parasympathetic activity via HRV can provide insights on physiological stress, with higher level of stress resulting in lower HRV. For example, in the context of sports, heavy training is responsible for shifting the cardiac autonomic balance toward a predominance of the sympathetic over the parasympathetic drive. This means that heavy training will typically reduce HRV. Therefore, by monitoring HRV we can hopefully adjust and optimize training, reduce the risk of overtraining and ultimately improve performance.
Your heart does not beat at a constant frequency. HRV analysis refers to different ways of quantifying this variability between consecutive beats.
Getting Your HRV Data
Up to a few years ago, HRV was used mainly by elite athletes and coaches or academic researchers working at the intersection of sports, fitness, health and medicine. Many of these experts were able to show links between HRV and performance as well as recovery or training load.
However, in the recent past many new, affordable and user-friendly tools have been developed. These tools typically rely on commercially available heart rate monitors (e.g. a Polar chest strap) to analyze data, compute HRV and provide guidance to the user.
The latest developments go even a step further. With apps like HRV4Training, HRV can be computed accurately without the need for any external sensor or device.
HRV4Training
HRV4Training uses the phone camera to extract photoplethysmography (PPG, basically blood flow from the finger) and then determine markers of the autonomous nervous system activity, in particular parasympathetic activity. The technique has been clinically validated and proved to be a reliable measurement, as good as standard electrocardiograms with sticky gel electrodes [3, 4, 5].
By quantifying parasympathetic activity the app is able to translate the information into an assessment of training load and provide actionable insights on physical condition, helping users to better understand how their body responds to trainings and other important factors in life (e.g. sleep, stress, etc.) [6, 7, 8, 9].
Comparison between RR intervals extracted from a chest-worn Polar H7 sensor and the iPhone Camera using the signal processing techniques implemented in HRV4Training. The data was collected during a paced breathing exercise. Increases and decreases in RR interval values due to inhaling and exhaling are clearly visible in both traces.
From Data to Insights
Even with the right tools, HRV can be difficult to gather under the right conditions, and especially interpret. Many factors influence HRV, from body posture to respiration, age, genetics, gender, physical exercise, chronic health conditions and more.
So how can we get reliable insights from HRV data, if it is affected by so many factors?
Get your Baseline
We do so in two ways. First, we need to look only at measurements with respect to ourselves, which means we need to collect a baseline, or a series of recordings so that the effect of different stressors is always evaluated with respect to what are our normal values, without looking much at the general population.
Secondly, we also need to control for as many of the previous factors as we can, and then evaluate the effect of what we care about. For example, in the context of optimizing performance, apps like HRV4Training provide a set of simple rules (or best practices) so that the measurements your take at home are as close as possible to supervised laboratory recordings, and your data is more reliable.
Best Practices
Here are a few best practices for short HRV measurements that are very important to follow to get consistent results that can be interpreted correctly:
- Take the measurement first thing after waking up, while still in bed. This way you have consistent time of the day, and you are not affected by other stressors (only exception is if you need to empty the bladder, in that case, do it, then go back, rest 1 or 2 minutes to make sure your body is not affected by physical activity, and take the measurement). Don’t ready your email before the measurement!
- Measurement duration: short measurements have been validated multiple times, and you can trust a 60 seconds recording for rMSSD (time domain feature).
- Breathing is key, it doesn’t really matter what breathing frequency you pick, but be consistent, use the same every time
- Body position: lying, sitting or standing are all good, again what matters is that you do always the same, and if you decide to stand, be patient, wait a minute or two before recording since your body needs to be at rest
Acute changes and long-term trends
HRV is typically analyzed in two ways; acute HRV changes and long-term trends. Acute changes refer to the easiest interpretation, i.e. on a day following intense training HRV is expected to drop. HRV4Training will provide you with daily advice using a slightly more complex interpretation of this principle.
While it is definitely interesting to look at HRV on a day to day variability basis, and understand the impact of intense training sessions, much research is now trying to use this data to understand more about our overall condition over longer periods of time, weeks to months, in the context of a training program [8, 9, 10].
Questions we can try to answer are: How are we adapting to a new training plan? is our physiological condition optimal while approaching a race or should we change something? Are we at risk of overtraining or accumulating fatigue?
From recent research is clear that HRV alone as traditionally analyzed, cannot answer all these questions. When we look at HRV over longer periods of time, the common rule, which is higher HRV equals better condition, does not necessarily hold anymore.
However, by combining a series of physiological parameters and methods we can definitely learn more about our physical condition.
By looking together at changes in baseline HRV, meaning our weekly averages over time, as well as looking at the variability within our HRV scores, for example if our readings are jumping around a lot during a week or are pretty much the same score every day, together with information about heart rate and of course our current training program, meaning training load, and intensity, there are some consistent patterns that we can identify.
Research shows that lower variability between readings together with a stable or increasing HRV baseline can be more representative of good adaptation to training, while the same reduction in variability between readings when associated with higher heart rate and lower HRV baseline is more representative of fatigue.
So in this context looking at multiple parameters can help better understand what is going on and tools like HRV4Training are constantly evolving to bring this analysis in the app and do the math for you, so that you can use the information to further optimize your trainings.
For questions / inquiries / feedback about HRV4Training, just drop a line to @marco_alt on Twitter or visit the HRV4Training blog.
References
[1] Sztajzel, Juan. “Heart rate variability: a noninvasive electrocardiographic method to measure the autonomic nervous system.” Swiss medical weekly 134 (2004): 514-522.
[2] Thayer, Julian F., Shelby S. Yamamoto, and Jos F. Brosschot. “The relationship of autonomic imbalance, heart rate variability and cardiovascular disease risk factors.” International journal of cardiology 141.2 (2010): 122-131.
[3] Russoniello, C. V., et al. “A measurement of electrocardiography and photoplethesmography in obese children.” Applied psychophysiology and biofeedback 35.3 (2010): 257-259.
[4] Lu, Sheng, et al. “Can photoplethysmography variability serve as an alternative approach to obtain heart rate variability information?.” Journal of clinical monitoring and computing 22.1 (2008): 23-29.
[5] HRV4Training Blog: http://www.hrv4training.com/blog/heart-rate-variability-using-the-phones-camera
[6] Garet, Martin, et al. “Individual interdependence between nocturnal ANS activity and performance in swimmers.” Medicine and science in sports and exercise 36 (2004): 2112-2118.
[7] Pichot, Vincent, et al. “Relation between heart rate variability and training load in middle-distance runners.” Medicine and science in sports and exercise 32.10 (2000): 1729-1736.
[8] Kiviniemi, Antti M., et al. “Endurance training guided individually by daily heart rate variability measurements.” European journal of applied physiology 101.6 (2007): 743-751
[9] Myllymäki, Tero, et al. “Effects of exercise intensity and duration on nocturnal heart rate variability and sleep quality.” European journal of applied physiology112.3 (2012): 801-809
[8] Plews, D. J., Laursen, P. B., Kilding, A. E., & Buchheit, M. (2012). Heart rate variability in elite triathletes, is variation in variability the key to effective training? A case comparison. European journal of applied physiology, 112(11), 3729-3741.
[9] Stanley, Jamie, Shaun D’Auria, and Martin Buchheit. “Cardiac Parasympathetic Activity and Race Performance: An Elite Triathlete Case Study.” IJSPP 10.4 (2015).
[10] Buchheit, M. (2014). Monitoring training status with HR measures: do all roads lead to Rome?. Frontiers in physiology, 5. Chicago